Understanding the Future of Autonomous Agents: A Deep Dive
Autonomous agents are becoming a critical component of the interaction between businesses and individuals with artificial intelligence. Agents, built on powerful language models, have transformed previously human-run tasks from simple recall to complex problem-solving.
What are Autonomous Agents?
Autonomous agents are sophisticated systems designed to operate independently, carrying out tasks and making decisions without the need for direct human oversight. At their core, these agents are self-governing, meaning they possess the capability to manage their own actions and responses based on the information available to them. Their self-governance is a key characteristic, allowing them to function effectively in a wide range of scenarios, from simple, routine operations to more complex, dynamic environments.
Components of Autonomous Agents:
Tools for Added Functionality:
Autonomous agents often integrate various tools and resources to extend their capabilities. Tools range from specialized software applications to hardware devices, allowing the agent to perform specific tasks more efficiently. For example, an autonomous agent in a smart home system might use sensors to monitor environmental conditions or utilize algorithms to optimize energy usage. The integration of tools enables the agent to perform actions that go beyond its basic programming, adapting to the needs of the environment or the task at hand.
Memory for Knowledge Retention:
Memory is a crucial component of autonomous agents, allowing them to retain and recall relevant information over time. This could include data about past interactions, learned patterns, or contextual information that influences future decisions. By retaining this knowledge, the agent can improve its performance, make better predictions, and adapt its behavior based on experience. For instance, an autonomous vehicle might remember specific routes, traffic patterns, or driver preferences, enabling it to navigate more effectively in the future.
Reflection and Self-Critique:
Advanced autonomous agents possess the ability to reflect on their actions and self-critique their performance. This means they can evaluate the outcomes of their decisions, identify areas for improvement, and adjust their strategies accordingly. Reflection might involve assessing the effectiveness of a chosen path, while self-critique could lead to modifications in decision-making algorithms or the adoption of alternative approaches. This reflective capability is essential for continuous learning and adaptation, especially in environments where conditions are constantly changing.
Adaptation to Dynamic Environments:
One of the most important aspects of autonomous agents is their ability to operate in dynamic, unpredictable environments. By processing real-time data and considering the context of their actions, these agents can make informed decisions that align with current conditions. For example, a robotic agent in a manufacturing setting might need to adjust its operations in response to machine malfunctions or changes in production requirements. The agent’s autonomy allows it to respond quickly and effectively, minimizing disruptions and maintaining efficiency.
Different Types of Agents
Autonomous agents are not monolithic; they come in various forms, each suited for different tasks:
Simple Reflex Agents: React to current conditions without considering historical data. (ex. Pura, Cubo)
Model-Based Agents: Maintain an internal state to make decisions based on past interactions. (ex. Octopus, Innit, AWAIR)
Goal-Based Agents: Focus on achieving specific goals, planning actions with future consequences in mind. (ex. Healios, Meditopia, Nurecover)
Utility-Based Agents: Aim for the best possible outcome by balancing likelihood and utility. (ex. Honor, FitXR)
Learning Agents: Continuously improve performance by adapting to new circumstances. (ex. Ginger, Alma)
Hierarchical Agents: Operate on multiple levels, using simpler agents as building blocks for more complex actions. (ex. SmartRent, Silvernest)
Key Characteristics of Autonomous Agents
For an agent to be truly autonomous, it must exhibit several key characteristics:
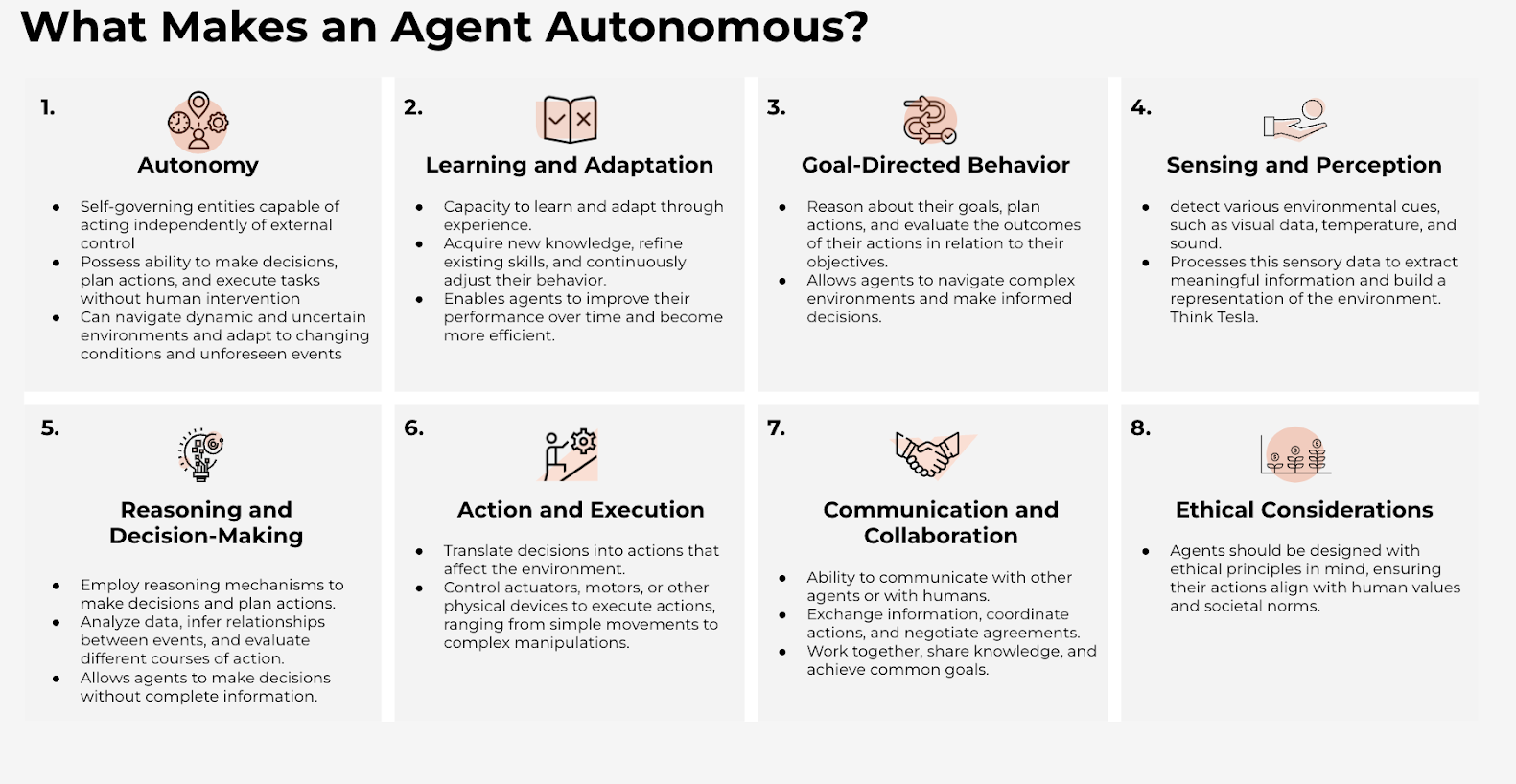
The Market Landscape for Autonomous Agents
The market for autonomous agents spans multiple layers, each with its own set of components and key players:
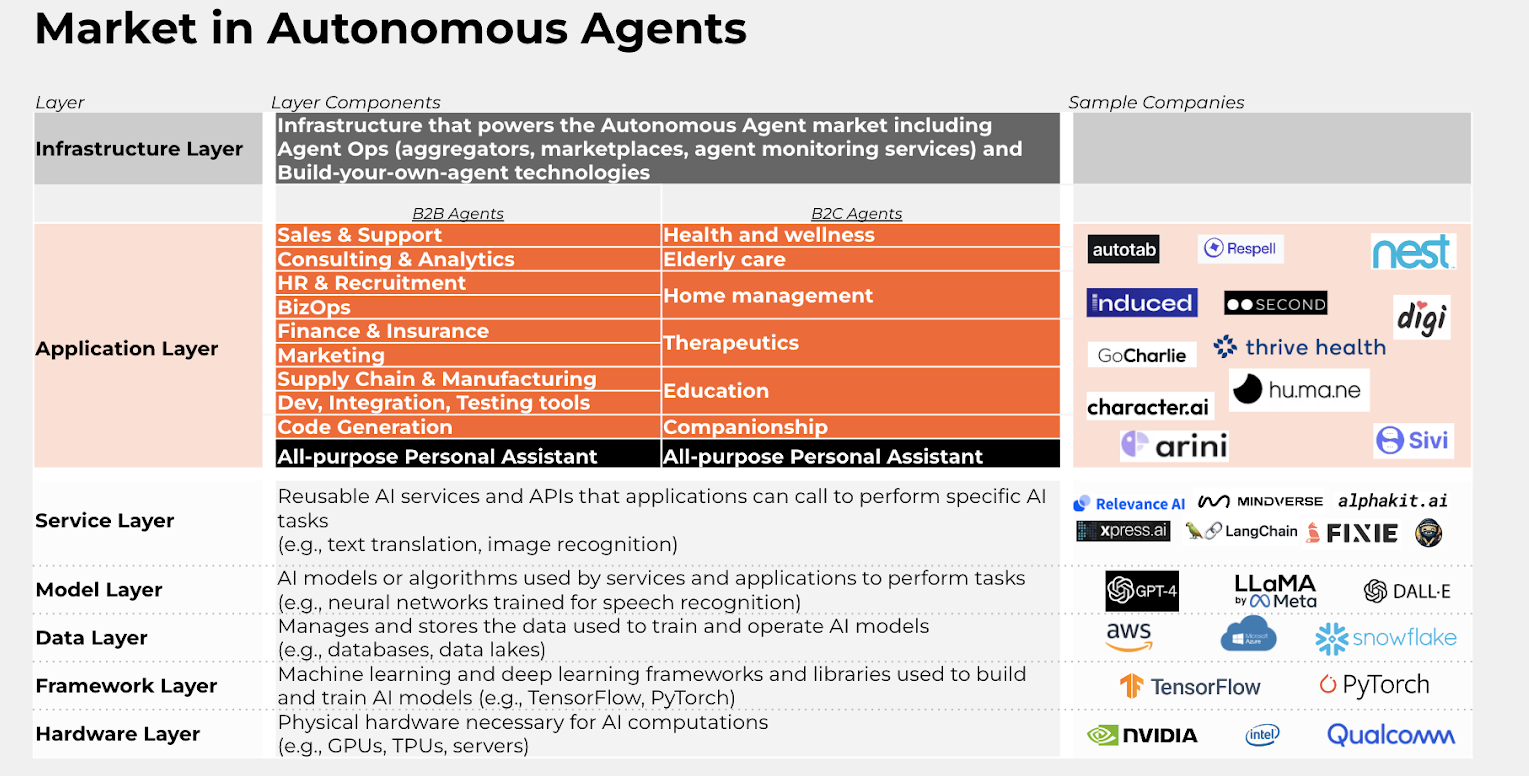
Application Layer: Industry-Specific Developments
Autonomous agents are also finding tailored applications in both B2B and B2C settings:
B2B Applications: Companies are deploying autonomous agents across multiple business functions such as sales, support, finance, and supply chain management. For instance, AI-driven analytics are enhancing sales forecasting, while predictive models are optimizing resource allocation in marketing and operations.
B2C Applications: In the consumer space, AI agents are becoming integral to daily life. From AI-powered smart speakers and home management systems to personalized health and wellness coaching, these agents are enhancing user convenience and improving quality of life.
Industry Use Cases
Autonomous agents are finding applications across various industries, including:
Healthcare
AI agents are being used for real-time data analysis, remote monitoring of elderly patients, and predictive analytics for preventive care.
Key trends in the industry point toward leveraging autonomous agents to drive innovation and efficiency. For instance:
Precision Medicine and Personalized Treatment: AI-driven analytics are transforming healthcare by enabling precision medicine. By analyzing vast amounts of patient data, including genetics, lifestyle, and medical history, AI systems can recommend personalized treatment plans that are more effective and tailored to individual patient needs.
Predictive Analytics for Disease Prevention: Agents are increasingly used for predictive analytics in healthcare, allowing for early detection and prevention of diseases. Agents can identify at-risk individuals and suggest preventive measures, thereby reducing the incidence and severity of diseases.
Enhanced Diagnostic Accuracy: AI tools are improving the accuracy of diagnostics by analyzing medical images, pathology reports, and other diagnostic data with greater precision than traditional methods. This leads to earlier and more accurate diagnosis of conditions such as cancer, heart disease, and neurological disorders. (ex. Butterfly Network
Streamlined Clinical Workflows: Agents are being integrated into various aspects of healthcare operations, such as administrative tasks, patient scheduling, and electronic health records management. This automation streamlines clinical workflows, reduces the burden on healthcare professionals, and allows them to focus more on patient care. (ex. Assort Health)
Enhanced Patient Education and Support: Agents are playing a growing role in patient education and support by providing tailored information and resources based on individual patient needs. Through interactive platforms, these agents offer guidance on medication adherence, lifestyle changes, and post-treatment care, empowering patients to manage their health more effectively and reducing the burden on healthcare providers.
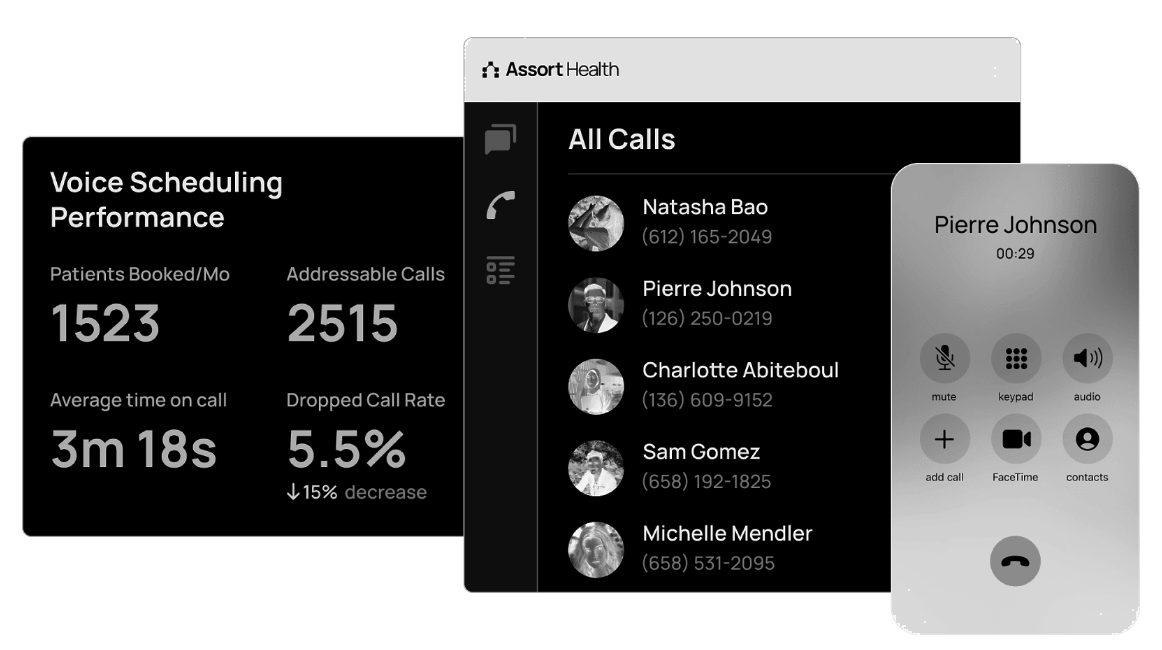
Assort Health Figures
Finance
Autonomous agents are playing a transformative role in the finance sector, with their ability to analyze large volumes of data and execute complex tasks with precision.
Some of the Key trends include:
Data-Driven Decision Making: AI-driven analytics are being extensively used for smarter financial forecasting, risk management, and investment strategies. These analytics enable financial institutions to make more informed decisions, based on real-time data, which improves overall efficiency and profitability. (ex. Yellow AI)
Enhanced Personalization: Autonomous agents in finance are increasingly used to tailor financial advice and services to individual clients, based on their unique financial history and goals. This level of personalization enhances customer satisfaction and loyalty, as clients receive advice that is more closely aligned with their needs.
Wider Integration Across Financial Functions: Beyond customer service, AI agents are being integrated into various financial functions such as compliance monitoring, fraud detection, and portfolio management. This holistic approach to AI deployment helps institutions streamline operations and reduce costs. (ex. skit.ai, a conversational agent used primarily in the accounting industry for task automation)
Shift Towards Predictive Analytics: The finance sector is witnessing a shift from traditional descriptive analytics to predictive analytics. AI agents are now used to forecast market trends, anticipate customer behavior, and manage risks proactively, thereby providing a competitive edge.
Regulatory Compliance and Reporting: Agents are increasingly being utilized to ensure regulatory compliance and streamline reporting processes in the financial sector. By automating the monitoring of transactions, generating reports, and ensuring adherence to complex regulatory requirements, agents reduce the risk of non-compliance and help institutions navigate evolving regulatory landscapes more efficiently
Sales & Support
As shown in other verticals, agents are proving arguably the most useful in the sales & support sector, for instance:
Personalized Customer Engagement: Agents are transforming sales and support by enabling personalized customer engagement. By analyzing customer data, including purchase history and behavior, agents can tailor interactions and recommendations to individual customers, resulting in higher satisfaction and increased sales. (ex. Liberate. Liberate’s agent is customizable and trainable to cater toward any type of business that uses voice agents for customer engagement. )
Enhanced Sales Forecasting: Agents are improving sales forecasting by analyzing market trends, customer behavior, and historical sales data. This allows sales teams to make more accurate predictions, optimize inventory levels, and allocate resources more effectively, ultimately boosting sales performance.
Automated Routine Tasks: Agents are being integrated into sales and support operations to automate routine tasks such as data entry, lead scoring, and follow-up communications. This automation frees up human agents to focus on higher-value activities, improving overall efficiency and productivity. (ex. Infer, a personalized voice agent that can be customized to help with an array of routine tasks)
Real-Time Customer Insights and Feedback: Agents are enhancing the ability to gather real-time customer insights and feedback. By continuously analyzing customer interactions and behaviors across various touchpoints, agents provide immediate insights that can be used to adjust sales strategies, improve product offerings, and refine support services.
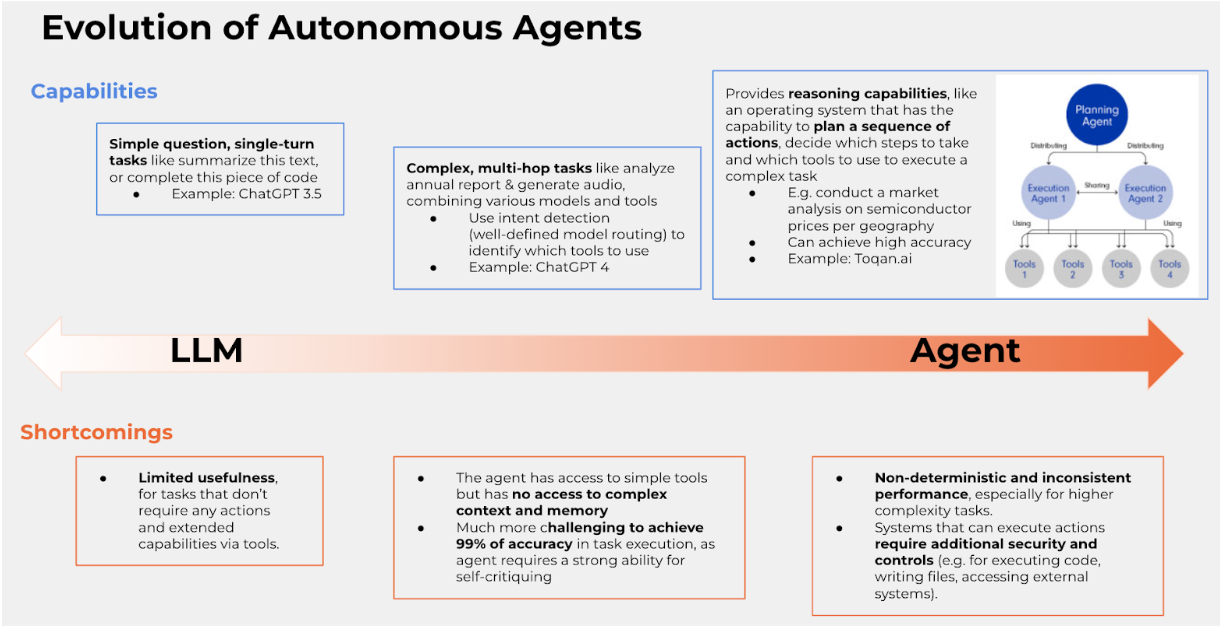
The Path Forward: Challenges and Opportunities
Despite their potential, autonomous agents face several challenges:
Reliability. The non-deterministic nature of language models means that the same query might produce different results, affecting reliability.
Scalability. Additionally, integrating these agents into existing systems, especially in enterprise environments, poses scalability and security challenges.
Non-deterministic nature of LLMs. Rephrasing a user request can result in different approaches/action plans to form final response, it then becomes clearer when agent has access to more tools, and executes more steps in between
Probabilistic nature of Gen AI: Certain use cases can only be unlocked once agent’s reache >99% of accuracy, it remains a challenge given the reliability of end-to-end agent workflows. Giving an agent the ability to reflect, self-critique and therefore the awareness of “being wrong” can be helpful
Limited short-term memory: This Reduces number of steps an agent can perform before forgetting insights from earlier steps, which are extending to ~500 steps
As technology matures and integration hurdles are overcome, autonomous agents will likely become more prevalent, offering tailored solutions across various verticals. The future of AI is one where these agents not only augment human capabilities but also operate independently, driving efficiency and innovation across industries.
The evolution of autonomous agents is a testament to the power and potential of AI. As agents become more sophisticated and integrated into both business and consumer applications, they will undoubtedly reshape how we interact with technology. By understanding their capabilities, challenges, and market landscape, we can better prepare for a future where autonomous agents play a central role in our daily lives and work environments.
————————
If you are a builder, investor or researcher in the space and would like to have a chat – please reach out to ayla.j@thelotuscapital.com or amit.k@thelotuscapital.com. We would love to hear from you!