The Future of Semiconductors: Trends Shaping Performance Computing and Data Infrastructure
Semis are the silent agent behind nearly every technological breakthrough this year, powering everything from AI-generated e-vites to Waymo rides to edge computing and more. In 2024, breakthroughs in high-performance computing, customized chip architectures, and intelligent workload distribution have redefined what’s possible across industries, setting the foundation for a more connected and intelligent world. Across advanced materials, graphene, gallium nitride (GaN), and silicon carbide (SiC) have enhanced chip performance and efficiency. Innovative fabrication techniques — such as Arieca‘s liquid metal elastomers — have shrunk node sizes and addressed heat dissipation challenges. In some case, chipmakers have brought innovation to infrastructure, for instance Fungible Systems (now Microsoft), which uses disaggregated systems to accelerate data-centric tasks in data centers.
While there is so much to unpack in the landscape, we at Lotus are most excited about innovations behind:
- High performance compute
- Customization and modularization
- Intelligent placement of compute
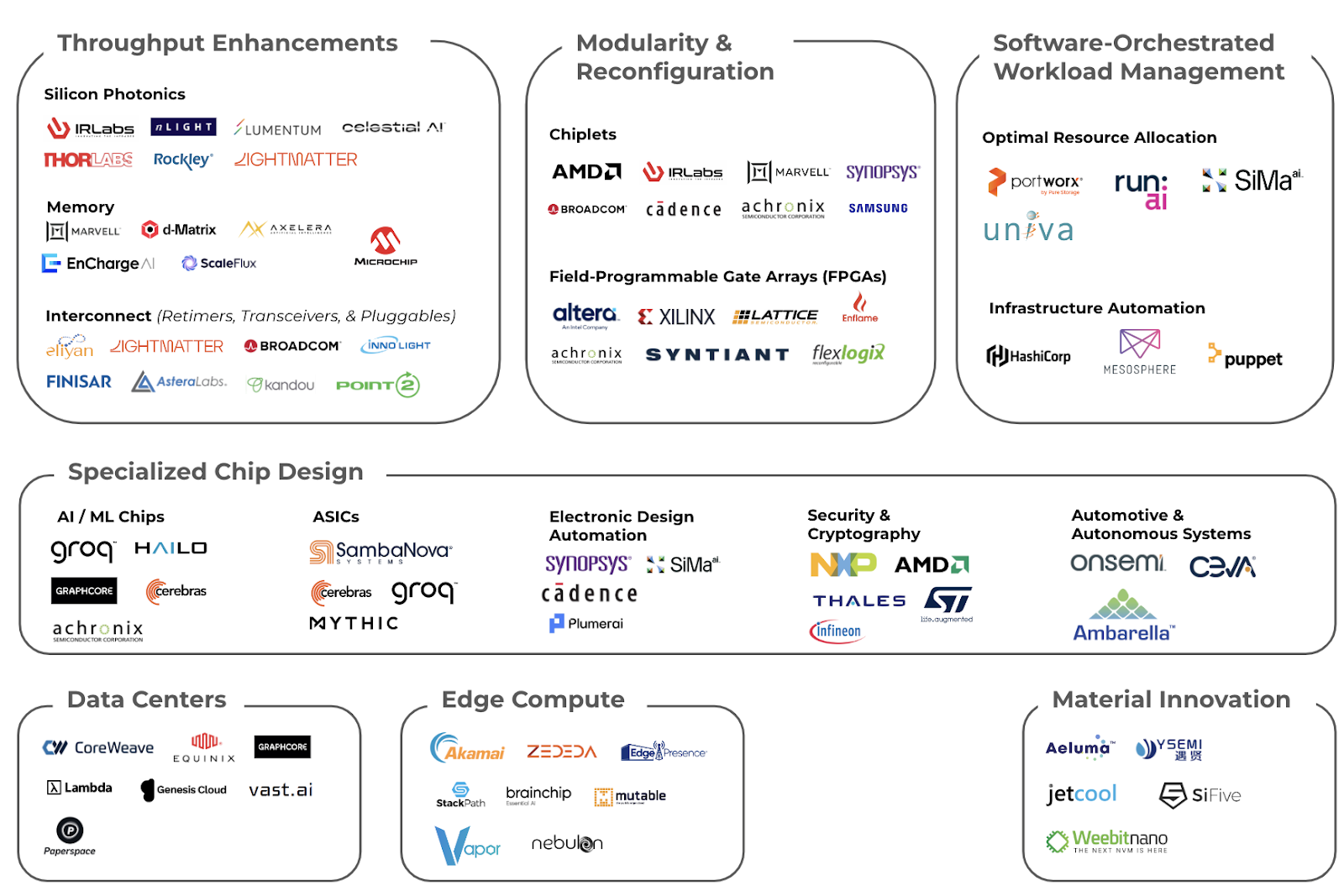
1) High-Performance Computing (HPC) Innovations
High-performance computing (HPC) enables faster processing, efficient resource utilization, and scalable infrastructure. Innovation happens within chips in a rack, between racks, and in the connections between chips.
Scale-Up Technologies amplify compute power within a rack. Scale-up technologies focus on maximizing performance within a single rack by improving interconnections, memory access, and compute integration. These technologies ensure the fastest data transfer and processing over short distances, enabling more efficient operations.
- Silicon Photonics: Silicon photonics facilitate data transmission at nearly the speed of light. This technology reduces latency and significantly increases bandwidth, making it ideal for high-speed intra-rack communication. Photonic Integrated Circuits (PICs) integrate optical components such as lasers, waveguides, and modulators onto a single chip. Unlike traditional electronic circuits that rely on electrons, PICs use photons for data transmission, enabling significantly reduced latency and power consumption. This innovation is particularly beneficial for bandwidth-intensive applications like AI and machine learning.
- Memory Stacking: Innovations in memory stacking integrate various configurations of DRAM and SRAM directly onto boards, providing faster data access while minimizing physical footprint. This approach is essential for memory-intensive applications in HPC.
- Chiplets: Chiplet technology involves integrating multiple smaller chip components into a cohesive package instead of relying on a monolithic chip design. Advanced interconnect technologies, such as high-speed interfaces and silicon bridges, allow these chiplets to deliver increased functionality and scalability while overcoming the limitations of traditional die scaling.
Scale-Out Technologies connect racks for distributed computing. Scale-out technologies expand computational capacity by connecting multiple racks, emphasizing distributed systems that enable scalability and flexibility. These systems typically rely on switches and high-speed protocols to ensure seamless communication between racks.
- Interconnect Technologies: High-speed communication protocols and hardware components enhance data transfer between distributed nodes, improving overall system efficiency. These technologies are crucial for scaling operations in data centers and supporting advanced semiconductor designs.
- Software-Orchestrated Workload Management: Software platforms optimize distributed systems by automating workload scheduling, execution, and monitoring. These solutions ensure scalability, resource efficiency, and reliability, particularly for enterprises managing large-scale data processing and cloud services. This segment can be broken down further into workload optimization solutions and infrastructure automation for multi-cloud environments.
- In-Memory Computing: Integrates processing and memory functions within the same hardware unit, significantly reducing data movement between the processor and memory. This approach, taken by Axelera AI and EnCharge AI, decreases latency and power consumption, making it particularly advantageous for AI and ML applications that require high-speed data processing
Retimers, Transceivers, and Pluggables connect things in a box. These mechanisms focus on throughput rather than networking algorithms. Components optimize high-speed data transmission, ensuring signal integrity and scalability in networking systems.
- Retimers: amplify and refine signals over long cables by optimizing light transmission, adjusting to narrower wavelength increments or using multiple colors to improve signal clarity. Retimers are neither scale-up nor scale-out technologies, but they serve as enabling infrastructure to optimize and extend performance of data transmission.
Tranceivers and pluggables work with retimers
- Transceivers: combine a transmitter (Tx) and a receiver (Rx) into one unit. They converts electrical signals into optical signals for transmission and vice versa for reception. They play a vital role in enabling efficient data communication across optical fibers.
- Pluggables: are a specific form factor of transceivers designed to be hot-swappable, meaning they can be easily inserted or removed from networking hardware like switches and routers without shutting down the system. They provide modular, scalable networking capabilities by allowing users to upgrade or replace optical modules as needed.
Transceivers and pluggable are primarily optical components for long-distance communication, while retimers are electrical components focused on maintaining signal integrity over shorter distances. They complement each other in building robust and scalable high-speed communication systems.
2) Customization in Chip Design
With widening industry needs, a growing diversity of workloads has created the need for specialized and modular chip designs. Customization and specialization represent a departure from prior generalized “one-size-fits-all” chips.
Specialized AI and ML semiconductors
These chips are uniquely designed to address the specific demands of AI and ML workloads, which differ significantly from traditional computing tasks. They are optimized for parallelism, high-speed data processing, and energy efficiency, enabling them to handle the vast computational needs of AI/ML applications. Specialized AI / ML chips include: GPUs (Graphics Processing Units), TPUs (Tensor Processing Units), NPUs (Neural Processing Units) and analog AI chips.
AI/ML semis can be further divided into:
- Training focused: Chips like those from Cerebras Systems, Graphcore, and Luminous are designed for intensive AI model training tasks.
- Inference focused: Solutions from Groq and Hailo cater to efficient AI inference in edge and cloud environments.
As models mature, the market expects that there will be a shift in demand away from training focused semis and towards inference focused chips
Application Specific Integrated Circuits
Application-Specific Integrated Circuits (ASICs) – such as Groq and Cerebras – are custom-designed chips tailored for specific applications or tasks, offering optimized performance, efficiency, and cost-effectiveness compared to general-purpose processors. Unlike general-purpose processors that handle a wide range of tasks, ASICs are engineered to perform a particular function or set of functions.
Field-Programmable Gate Arrays (FPGAs)
Reconfigurable chips, such as Flex Logix and Syntiant – allow customization post-manufacturing, catering to diverse and evolving computational needs. FPGAs can be programmed multiple times to adapt to new tasks or optimize performance. Unlike ASICs, which are designed for one fixed function, FPGAs are flexible and can be updated or reprogrammed as requirements change.
Modular chip design
Often referred to as chiplet technology, modular chips are an approach in semiconductor engineering where a system-on-chip (SoC) is constructed by integrating multiple smaller, specialized chips—known as chiplets—within a single package. Each chiplet performs a specific function, and their combination allows for the creation of complex, high-performance systems. This method contrasts with traditional monolithic chip designs, where all functions are integrated onto a single, large silicon die.
AI-powered chip design
Involves training models on vast datasets of existing chip designs and performance metrics. These models learn to predict the outcomes of various design choices and can generate new designs that meet specified criteria. AI models – such as Flex Logix, Syntiant, and Enflame – are accelerating chip design by optimizing layouts and configurations, reducing development cycles, and enhancing performance.
3) Intelligent placement of compute
Distributed Compute Ecosystems
Merging data center and edge computing into a unified framework emphasizes the concept of distributed compute ecosystems, where workloads are dynamically managed based on location and application requirements. The key is to think of them as parts of a continuum of compute rather than isolated silos. Conceptually, the merge works as follows: Compute resources span across central data centers, regional facilities, and edge nodes. Workloads are distributed dynamically based on latency, bandwidth, and processing requirements.
Data Centers
Centralized facilities, such as those operated by CoreWeave and Lambda, are optimized for large-scale batch processing, analytics, and storage. Often located far from end-users, the advantages of data center innovations include scalability, cost-efficiency, and high-density computing.
Edge Computing
Decentralized, smaller compute nodes – such as Vapor IO, Mutable, and EdgePresence – are positioned closer to end-users for low-latency tasks. Within edge computing innovation, low-power processors are chips designed for edge devices, balancing performance with energy efficiency. Integrated connectivity solutions are semiconductors that facilitate seamless communication between IoT devices and central systems. Edge computing wins for its real-time responsiveness, reduced bandwidth usage, and ability to maintain local data privacy.
Bridging the Two
Regional Hubs or Mid-Tier Data Centers, such as AWS Local Zones, serve as intermediaries, enabling a hierarchical compute structure that balances latency and capacity.
—————————————–
If you are a builder, investor or researcher in the space and would like to have a chat – please reach out to me at ayla@thelotuscapital.com